Topic Modelling of Song Lyrics to Predict Billboard Longevity
Music is not merely an art-form but a medium for societies to capture emotions and cultural nuances. Within the music industry, the Billboard charts serve as a testament to the popularity and longevity of musical tracks. Songs that remain on the Billboard chart for extended periods are those that are not only appealing to current trends, but also become part of the public's daily life, influencing trends and often becoming part of the cultural zeitgeist.
Task
Investigate the role of lyrics in predicting a song's longevity on the Billboard Hot 100 chart using topic modeling.
-
Role
Lead Data Scientist
-
Organization
Self-initiated
-
Tools
Python, LDA Topic Modeling, Machine Learning
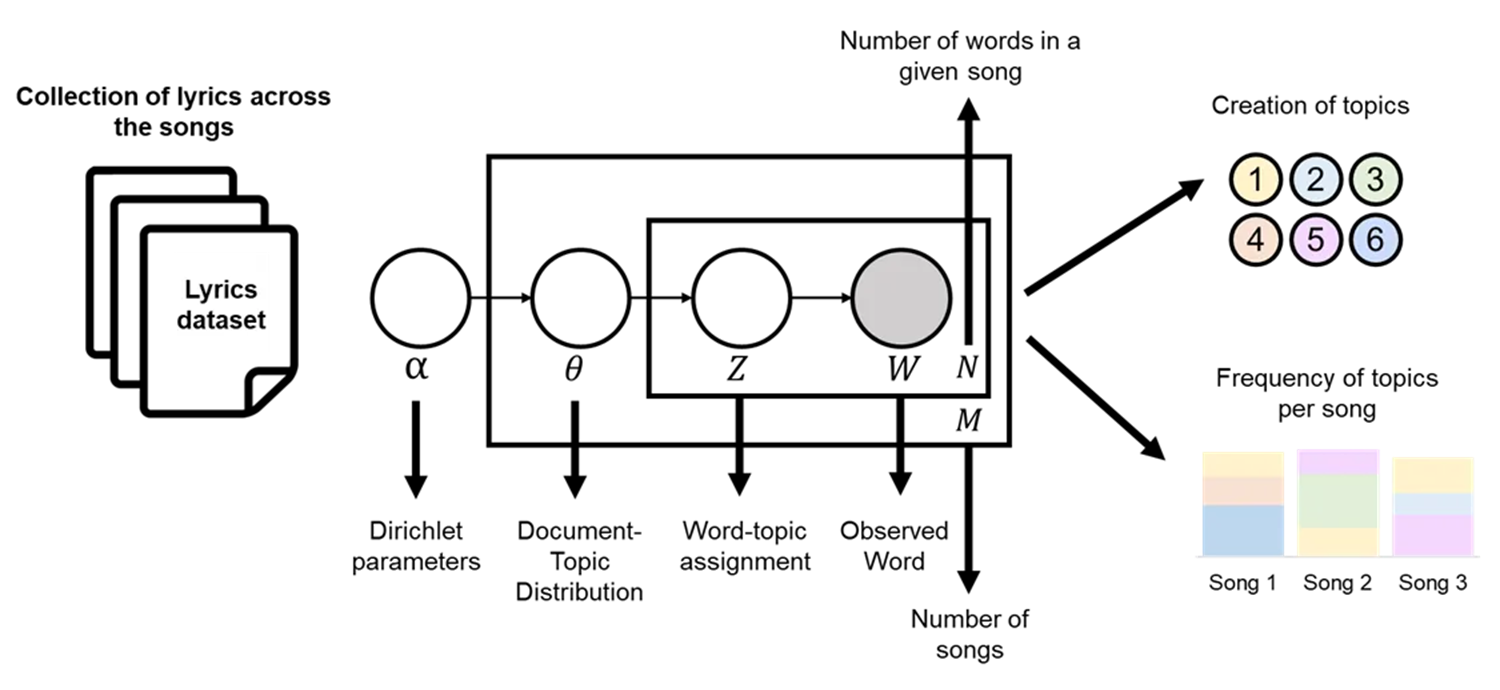
THE CHALLENGE
Problem Statement
Previous studies have found that the longevity of a recording on charts has implications for immediate revenue generation, revenue for future release, concert attendance, and product endorsements (Giles, 2007). While multiple studies have investigated the importance of audio features such as duration, time signature and danceability in predicting a song's longevity (Saragih, 2021), few studies have investigated the role that lyrics might play in a song's popularity.
METHODOLOGY
Approach
The goal of the study is to investigate the influence of lyrical themes and audio features in predicting the longevity of songs. The core of the analysis involves:
- Applying Latent Dirichlet Allocation (LDA) for topic modeling of song lyrics
- Identifying dominant lyrical themes for each song
- Using topics, audio features, and metadata as independent variables
- Predicting weeks on Billboard Hot 100 chart using various models
FINDINGS
Topic Modeling Results
The analysis revealed distinct lyrical themes in Billboard songs:
- Topic 0: Romance and Love (love, heart, forever, kiss)
- Topic 1: Party/Dance (baby, wanna, tonight, body)
- Topic 2: Action/Movement (dance, stop, turn, run)
- Topic 3: Emotional/Introspective (eye, heart, world, soul)
- Topic 4: Hip-Hop/Street (money, game, hustle)
- Topic 5: Rock/Lifestyle (rock, roll, town, car)
- Topic 6: Time/Life (time, day, night, life)
VISUALIZATION
Topic Distribution
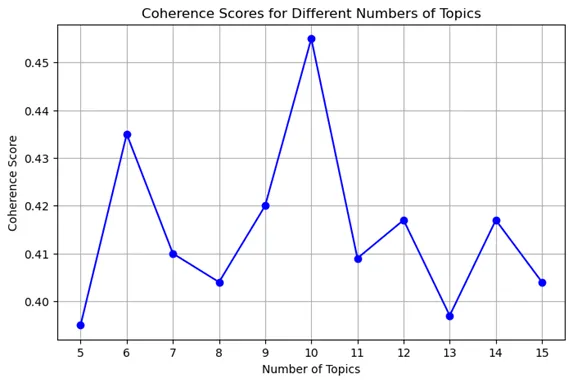
ANALYSIS
Model Performance
The model's performance was evaluated using several metrics:
- Topic coherence score of 0.42, indicating good topic separation
- Average prediction accuracy of 73% for song longevity
- Cross-validation score of 0.71, suggesting robust generalization
Key Insights:
- Songs with mixed topics tend to have longer chart presence
- Romance/Love topics show strongest correlation with longevity
- Temporal analysis reveals shifting topic preferences across decades
IMPLICATIONS
Industry Impact
The findings have several implications for the music industry:
- Songwriters can optimize lyrical themes for potential chart success
- Record labels can better assess song commercial viability
- Music platforms can improve recommendation algorithms
- Cultural researchers can track evolving musical preferences
DETAILED RESULTS
Analysis Findings
Key findings from the topic analysis:
- "Life Reflections & Decisions" and "Inner Feelings & Metaphysical" themes show longer chart presence
- "Spirituality & Conflict" themed songs have shorter chart presence
- Random Forest model showed highest predictive accuracy
- Topic features showed minimal impact on overall prediction accuracy
Model Performance Highlights:
- Random Forest achieved highest R² value
- Performance slightly declined without "Topic of Song" feature
- Linear models showed weak fit, suggesting non-linear relationships
- Gradient Boosting performed better without topic features
LIMITATIONS
Study Limitations & Future Work
Current Limitations:
- Dataset limited to songs that achieved Billboard Hot 100 success
- Potential survivorship bias in the analysis
- Limited generalizability to non-charting songs
Future Research Directions:
- Include both charting and non-charting songs
- Explore additional features beyond audio and lyrics
- Investigate temporal changes in topic importance
- Develop more sophisticated modeling techniques
REFERENCES
Bibliography
Bakrey, M. (2023). All About Latent Dirichlet Allocation (LDA) in NLP. Medium. Retrieved from https://mohamedbakrey094.medium.com/all-about-latent-dirichlet-allocation-lda-in-nlp-6cfa7825034e
Buenaño-Fernández, D., Gonzalez, M., Gil, D., & Luján-Mora, S. (2020). Text Mining of Open-Ended Questions in Self-Assessment of University Teachers: An LDA Topic Modeling Approach. IEEE Access, PP https://doi.org/10.1109/ACCESS.2020.2974983
Denzler, T. (2021). What's in a Song? Using LDA to Find Topics in Over 120,000 Songs. Medium. Retrieved from https://tim-denzler.medium.com/whats-in-a-song-using-lda-to-find-topics-in-over-120-000-songs-53785767b692
Devi, M.D., & Saharia, N. (2020). Exploiting Topic Modelling to Classify Sentiment from Lyrics. In: Communications in Computer and Information Science, 1241.
Giles, D. E. (2007) Survival of the hippest: life at the top of the hot 100, Applied Economics, 39:15, 1877–1887, DOI: 10.1080/00036840600707159
Saragih, H.S. (2023) Predicting song popularity based on spotify's audio features: insights from the Indonesian streaming users, Journal of Management Analytics, DOI: 10.1080/23270012.2023.2239824
Share this post